3 ways to describe populations and samples
A population is a collection of measurements that you are interested in studying. For example, a population could consist of the incomes of the residents of the United States. Because analyzing every element of a population is often impractical, a sample of data may be chosen from a population and used as a substitute for the population.
A sample may be chosen in many different ways. It is extremely important to ensure that the members of a sample truly reflect the underlying population.
Measures of central tendency
In statistics, the mean, median, and mode are known as measures of central tendency; they are used to identify the center of a data set:
-
Mean: The average value of a data set.
-
Median: The value that divides a data set in half so that half the observations are less than the median and half are greater than the median.
-
Mode: The value that occurs most frequently in a data set.
The mean of a sample is computed as:
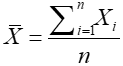
The mean of a population is computed as:
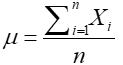
The median of a sample or a population is computed by sorting the data from the smallest value to the largest value and then finding the observation in the center — the value at which half of the observations in the data set are smaller than this value and half of the observations in the data set are greater than this value.
With an odd number of observations, you have one unique value in the center. With an even number of observations, you must average the two center values.
The mode of a sample or a population is computed by determining the value that occurs most frequently in the data set. Note that there can be any number of modes or there may be no mode at all.
Measures of dispersion
Measures of dispersion are used to find out how spread out the elements of a data set are. Three of the most commonly used measures of dispersion are:
- Range
- Variance
- Standard deviation
The range is equal to the difference between the largest and smallest values in a sample or a population.
The variance measures the average squared difference between the elements of a sample or a population and the mean. The formulas for computing the sample and population variances are slightly different.
The variance of a sample is computed as:
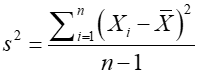
The variance of a population is computed as:
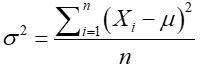
The standard deviation is the square root of the variance; it has the advantage that it is measured in the same units as the underlying data, while variance is measured in squared units.
The standard deviation of a sample is computed as:
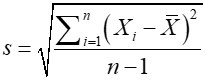
The standard deviation of a population is computed as:
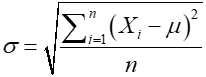
Measures of association
Measures of association are used to determine how closely two variables are related to each other. Two of the most commonly used measures of association are:
- covariance
- correlation
For both measures, a positive value indicates that two variables tend to move in the same direction, while a negative value indicates that two variables tend to move in opposite directions. A value of zero indicates that two variables are unrelated to each other (they are independent of each other).
The formulas for the sample covariance and population covariance are slightly different.
The sample covariance is computed as:
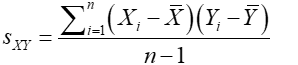
The population covariance is computed as:
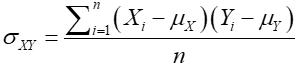
The correlation has more convenient properties than the covariance. Unlike the covariance, which has no maximum or minimum value, the correlation coefficient always has a value between –1 and 1. This makes it easier to intuitively understand both the strength and direction of the relationship between two variables with the correlation coefficient. Further, the correlation coefficient is unit-free — it is just a pure number. The value of the covariance is influenced by the units in which the sample or population data are measured, which is highly inconvenient.
The sample and population correlation coefficients both have the same value; although, their formulas use different notation.
The sample correlation coefficient is computed as:
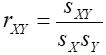
The population correlation coefficient is computed as:
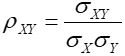
Random variables and probability distributions
A random variable is used to assign numerical values to all the possible outcomes of a random experiment. A probability distribution assigns probabilities to these numerical values. The two basic types of probability distributions are:
- Discrete
- Continuous
With a discrete probability distribution, there is only a finite number of possible values; with a continuous probability distribution, the number of possible values is infinite.
Discrete probability distributions
Two of the most widely used discrete probability distributions are:
- Binomial
- Poisson
The binomial distribution is based on a random process in which a series of independent trials take place. On each trial, only two possible outcomes can occur. The probability of a given number of successful outcomes during a fixed number of trials is:

The Poisson distribution is used to measure the probability that a specified number of events will occur over the next interval of time. Probabilities for the Poisson distribution are computed as follows:
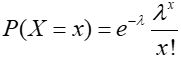
Continuous probability distributions
Some of the most widely used continuous probability distributions are:
- Uniform
- Normal
- Student’s t
- Chi-square
- F
The uniform distribution is used to describe a situation in which all possible outcomes of a random experiment are equally likely to occur. For a uniform random variable X that is defined over the interval (a, b) the equation is:
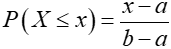
The normal distribution is one of the most widely used distributions in all applied disciplines such as economics, finance, psychology, biology, and so on. The normal distribution has several key properties, such as:
- It is described by a bell-shaped curve.
- It is symmetrical about the mean.
- It is defined over all real numbers between negative and positive infinity.
Normal probabilities are used for sampling distributions, confidence intervals, hypothesis testing, regression analysis, and many other applications. Normal probabilities can be computed with tables, with the Texas Instruments TI-84 calculator, Microsoft Excel, or a statistical programming language.
The student’s t-distribution (also known as the t-distribution) is often used in place of the normal distribution for cases when small samples are used. It is used for confidence intervals, hypothesis testing, regression analysis, and so on. The t-distribution has many properties in common with the standard normal distribution (where the mean is zero and the standard deviation is one), but it has a larger standard deviation and is characterized by degrees of freedom.
The chi-square distribution is a continuous distribution derived from the standard normal distribution. Unlike the standard normal distribution, it is positively skewed and only defined for positive values. The chi-square distribution can be used to test hypotheses about the population variance, for goodness of fit tests, and many other applications.
The F-distribution is another positively skewed continuous distribution. It is useful for testing hypotheses about the equality of two population variances and is also used in conjunction with analysis of variance (ANOVA) and regression analysis.
Understand sampling distributions
A sample statistic such as (the sample mean) can be thought of as a random variable that has its own probability distribution. According to the Central Limit Theorem, the sampling distribution of is normally distributed if the underlying population is normally distributed and/or the sample size is at least 30.
The mean of the sampling distribution of is:

The standard deviation of the sampling distribution of (known as the standard error of ) depends on the sample size relative to the population size:
- If the sample size is less than or equal to 5 percent of the population size, the sample is small, relative to the size of the population. In this case, the standard error is:
- If the sample size is greater than 5 percent of the population size, the sample is large, relative to the size of the population. In this case, the standard error is:
The term

How to construct confidence intervals
A confidence interval is a range of numbers that’s likely to contain the true value of an unknown population parameter, such as the population mean or proportion. When constructing confidence intervals for the population mean, there are two possible cases:
- The population standard deviation is known.
- The population standard deviation is unknown.
When the population standard deviation is known, the appropriate form for a confidence interval about the population mean is:
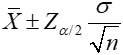
When the population standard deviation is unknown, the appropriate form for a confidence interval about the population mean is:
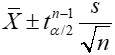
Explore hypothesis testing in business statistics
Hypothesis testing is carried out to draw conclusions about a population parameter such as the mean, proportion, variance, and so on. Hypothesis testing is a six-step procedure:
- Null hypothesis
- Alternative hypothesis
- Level of significance
- Test statistic
- Critical value(s)
- Decision rule
The null hypothesis is a statement that is assumed to be true unless there is strong contrary evidence. The alternative hypothesis is a statement that is accepted in place of the null hypothesis if there is sufficient evidence to reject the null hypothesis.
The level of significance is the probability of incorrectly rejecting the null hypothesis (this is referred to as a Type I Error). A test statistic is a numerical measure that is constructed to determine whether the null hypothesis should be rejected.
The critical value (or values) shows how extreme the test statistic must be in order to reject the null hypothesis. The decision as to whether or not to reject the null hypothesis is based on a comparison of the test statistic and the critical value (or values).
When testing hypotheses about the population mean, there are two cases:
- The population standard deviation is known.
- The population standard deviation is unknown.
When the population standard deviation is known, the test statistic and critical value (or values) are based on the standard normal (Z) distribution.
The test statistic is:
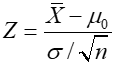
The critical values depend on the alternative hypothesis chosen:
Two-tailed test:

Right-tailed test:

Left-tailed test:

When the population standard deviation is unknown, the test statistic and critical value (or values) are based on the t-distribution.
The test statistic is:
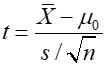
The critical values depend on the alternative hypothesis chosen:
Two-tailed test:

Right-tailed test:

Left-tailed test:

How businesses use regression analysis statistics
Regression analysis is a statistical methodology that helps you estimate the strength and direction of the relationship between two or more variables. Simple regression is based on the relationship between a dependent variable and a single independent variable, while multiple regression is based on the relationship between a dependent variable and two or more independent variables.
For simple regression analysis, the population regression line is:

The corresponding sample regression line is:
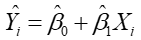
The results of regression analysis must be tested to ensure that the results are valid. One of the most important tests is known as the t-test; this is a hypothesis test that is used to determine if the slope coefficient equals zero. If this hypothesis cannot be rejected, it means that the independent variable (X) does not explain the value of the dependent variable (Y).
An important measure that is used to determine how well the regression model fits the sample data is known as the coefficient of determination (R2). The closer this measure is to one, the better is the fit of the sample regression line to the sample data.