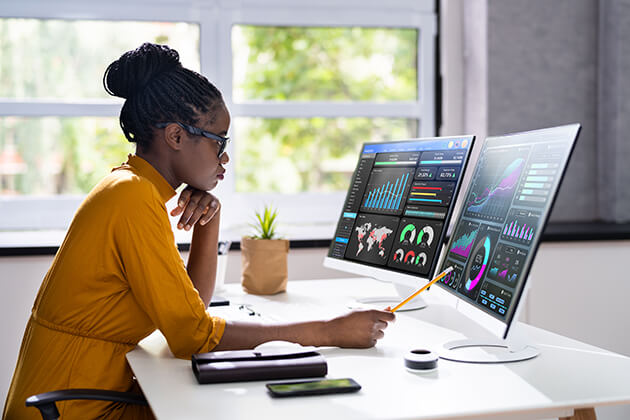
The legal department requires data to be handled by everyone in a manner consistent with laws and regulations. Product designers want data to inform creative decisions that align with marketplace demands and customer trends. Security professionals are tasked with ensuring that the data is appropriately protected.
Undoubtedly, a wide range of stakeholders want to harness the remarkable power of data.
To achieve these and other increasingly common business demands, you need some form of data control and accountability in your enterprise. Quality results require the diligent management of your organization’s data.
Data governance is all about managing data well.
Well-managed data can drive growth
Today, when data is managed well, it can drive innovation and growth and can be an enterprise’s most abundant and important lever for success.Well managed data can be transformational, and it can support the desirable qualities of a data-driven culture. This is when decisions at all levels of the organization are made using data in an informed and structured manner such that they deliver better outcomes internally and to customers.
Research confirms that most business leaders today want their organizations to be data-driven, but, according to a survey by NewVantage Partners, only around 32 percent are achieving that goal.
Successful data governance also means that data risks can be minimized, and data compliance and regulatory requirements can be met with ease. This can bring important comfort to business leaders who, in some jurisdictions, can now be personally liable for issues arising from poor data management.
Every organization manages data at some level. All businesses generate, process, use, and store data as a result of their daily operations. But there’s a huge difference between businesses that casually manage data and those that consider data to be a valuable asset and treat it accordingly. This difference is characterized by the degree in which there are formalities in managing data.
Broadly, the discipline in which an organization acts in recognition of the value of its information assets (a fancy term for data with specific value to an organization, such as a customer or product record) is called enterprise information management (EIM). Governing and managing data well is a central enabler of EIM, which also includes using technologies and processes to elevate data to be a shared enterprise asset.Data governance versus data management
Within the EIM space there are many terms that sound like they might mean the same thing. There is often confusion about the difference between data governance and data management. Data governance is focused on roles and responsibilities, policies, definitions, metrics, and the lifecycle of data.Data management is the technical implementation of data governance. For example, databases, data warehouses and lakes, application programming interfaces (APIs), analytics software, encryption, data crunching, and architectural design and implementation are all data management features and functions.
Data governance versus information governance
Similarly, in EIM, you may want clarity on the difference between data governance and information governance. Data governance generally focuses on data, independent of its meaning. For example, you may want to govern the security of patient data and staff data from a policy and process perspective, despite their differences. The interest here is on the data, not as much on the business context.Information governance is entirely concerned with the meaning of the data and its relationship in terms of outcomes and value to the organization, customers, and other stakeholders.
You might experience obvious overlap between the two terms. For sure, as a data governance practitioner, to some extent you’ll be operating in both the data and information governance worlds each day. This shouldn’t present an issue as long as the strategy for data governance is well understood.
My view is that understanding the context of data, a concept known as data intelligence, and the desired business outcomes, complement data governance efforts in a valuable manner.
The value of data governance
If an organization considers data to be a priority and it puts in place processes and policies to leverage the data’s value and reduce data risks, that organization is demonstrating a strong commitment to data controls and accountabilities. In other words, that organization values data governance.An increasing number of businesses value data governance; in fact, according to Anmut, a data consultancy, 91 percent of business leaders say that data is a critical part of their organization’s success.
Fundamentally, data governance is driven by a desire to increase the value of data and reduce the risks associated with it. It forces a leap from an ad hoc approach to data to one that is strategic in nature.
Some of the main advantages achieved by good data governance include:
- Improved data quality
- Expanded data value
- Increased data compliance
- Improved data-driven decision-making
- Enhanced business performance
- Greater sharing and use of data across the enterprise and externally
- Increased data availability and accessibility
- Improved data search
- Reduced risks from data-related issues
- Reduced data management costs
- Established rules for handling data
In the absence of formalized data governance, organizations will continue to struggle in achieving these advantages and may, in fact, suffer negative consequences. For example, poor quality data that is not current, inaccurate, and incomplete can lead to operating inefficiencies and poor decision-making.
Data governance does not emerge by chance. It’s a choice and requires organizational commitment and investment.