Just as the SE (standard error) formulas depend on what kind of sample statistic you're dealing with (whether you're measuring or counting something or getting it from a regression program or from some other calculation), confidence intervals (CIs) are calculated in different ways depending on how you obtain the sample statistic.
Suppose you study 25 adult diabetics (N = 25) and find that they have an average fasting blood glucose level of 130 mg/dL with a standard deviation (SD) of ± 40 mg/dL. What is the 95 percent confidence interval around that 130 mg/dL estimated mean?
To calculate the confidence limits around a mean using the formulas for large samples, you first calculate the standard error of the mean (SEM), which is
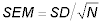
where SD is the standard deviation of the N individual values. So for the glucose example, the SE of the mean is
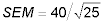
which is equal to 40/5, or 8 mg/dL.
Using k = 1.95 for a 95 percent confidence level, the lower and upper confidence limits around the mean are
CLL = 130 – 1.96×8 = 114.3
CLU = 130 + 1.96×8 = 145.7
You report your result this way: mean glucose = 130 mg/dL, 95%CI = 114–146 mg/dL. (Don't report numbers to more decimal places than their precision warrants. In this example, the digits after the decimal point are practically meaningless, so the numbers are rounded off.)
A more accurate version of the formulas for large samples uses k values derived from a table of critical values of the Student t distribution. You need to know the number of degrees of freedom, which, for a mean value, is always equal to N – 1.
Using a Student t table or a web page like StatPages, you can find that the Student-based k value for a 95 percent confidence level and 24 degrees of freedom is equal to 2.06, a little bit larger than the normal-based k value.
Using this k value instead of 1.96, you can calculate the 95 percent confidence limits as 113.52 and 146.48, which happen to round off to the same whole numbers as the normal-based confidence limits. Generally you don't have to use the more-complicated Student-based k values unless N is quite small (say, less than 10).
What if your original numbers (the ones being averaged) aren't normally distributed? You shouldn't just blindly apply the normal-based CI formulas for non-normally distributed data. If you know that your data is log-normally distributed (a very common type of non-normality), you can do the following:
Take the logarithm of every individual subject's value.
Find the mean, SD, and SEM of these logarithms.
Use the normal-based formulas to get the confidence limits (CLs) around the mean of the logarithms.
Calculate the antilogarithm of the mean of the logs.
The result is the geometric mean of the original values.
Calculate the antilogarithms of the lower and upper CLs.
These are the lower and upper CLs around the geometric mean.