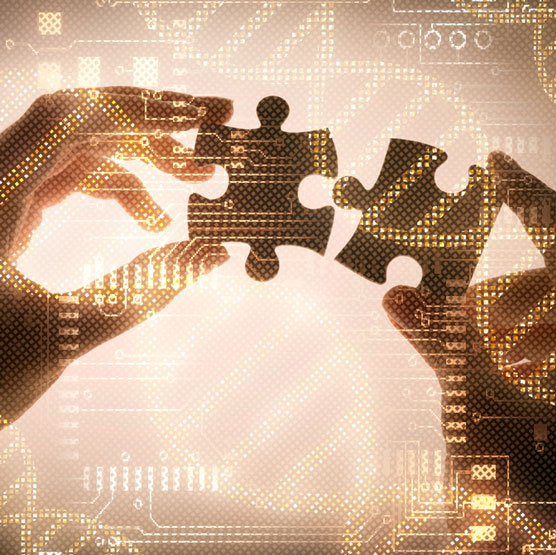
4 Types of AI Analytics
- Descriptive analytics = information, reveal what happened
- Diagnostic analytics = hindsight, reveal why something happened
- Predictive analytics = insight, present what will likely happen next
- Prescriptive analytics = foresight, recommend what to do next
4 Types of AI Modeling
- Association: Determines the probability that two contemporaneous events are related. For example, in sales transactions the association function can uncover purchase patterns, such as when a customer who buys milk also buys cereal.
- Classification: Reveals patterns that can be used to categorize an item. For example, weather prediction depends on identifying patterns in weather conditions (such as rising or dropping air pressure) to predict whether it will be sunny or cloudy.
- Clustering: Organizes data by identifying similarities and grouping elements into clusters to reveal new information. One example is segmenting customers by gender, marital status, or neighborhood.
- Regression: Predicts a numeric value depending on the variables in a given dataset. For example, the price of a used car can be determined by analyzing its age, mileage, condition, optional packages, and other variables.
4 Enabling Technologies for AI
- Computing
- Algorithms
- Data
- Storage
4 Vs of Big Data
- Volume
- Variety
- Velocity
- Visualization
4 Types of Visualization
Type | Use |
Comparison | Compare two or more values on an XY axis.
Examples: timeline, trend, ranking Types: line, column, bar, timeline |
Composition | Show how the parts relate to the whole.
Examples: revenue of product mix over time, breakdown of demographic data across the range of a variable Types: stacked bars/columns, pie/donut, stacked area, waterfall, polar |
Distribution | Show the value of one variable tracked across a set of categories.
Examples: sales across regions or stores, age ranges in demographic Types: histogram, line, area, scatter plot, map |
Relationship | Show the connection between two or more variables.
Examples: track revenue versus cost across regions or stores, show traffic or accident incidents by weather or time of day Types: scatter, bubble, line |
Text Mining versus Data Mining
Data mining | Text mining | |
Overview | Data mining searches for patterns and relationships in structured data. | Text mining transforms unstructured textual data into structured information to enable data analysis. |
Data type | Structured data from large datasets is found in systems such as databases, spreadsheets, ERP, and accounting applications. | Unstructured textual data is found in emails, documents, presentations, videos, file shares, social media, and the Internet. |
Data retrieval | Structured data is homogenous and organized, making it easy to retrieve. | Unstructured textual data comes in many different formats and content types located in a more diverse range of applications and systems. |
Data preparation | Structured data is formal and formatted, facilitating the process of ingesting data into analytical models. | Linguistic and statistical techniques — including NLP keywording and meta-tagging — must be applied to turn unstructured into usable structured data. |
Taxonomy | There is no need to create an overriding taxonomy. | A global taxonomy must be applied to organize the data into a common framework. |
Machine Learning
Learning phase
- Sample historical data (machine activity, customer attributes, transactions).
- Apply algorithm to historical data to learn key patterns and trends.
- Generate a model or set of rules or instructions.
Prediction phase
- Load the existing model.
- Apply the model to new data.
- Predict the likelihood of an outcome (such as customer churn).
The output of the prediction phase feeds back into the input of the learning phase to refine the model.
Classification Types
- Supervised classification depends on humans to label the training data that the model uses to classify new content.
- Unsupervised classification uses algorithms to discover relationships in the data and create categories without human intervention.
Drilling Down from AI to ML to DL
Technique | Description | Example |
Artificial Intelligence | Computing systems capable of performing tasks that humans are very good at | Recognize objects, recognize and make sense of speech, self-driving cars |
Machine learning | Field of AI that learns from historical data toward an end goal or outcome | Predict customers likely to churn |
Deep learning | Powerful set of machine learning techniques that mimic the brain’s neuron activities | Computer vision, colorize photos, deep fakes, mastering a game |
Data Science Project Methodology
Define the task | What problem or question do you want to address with data? |
Collect data | What data do you have that could answer our questions? |
Prepare the data | What do you need to do to prepare the data for mining? |
Refine the model | How can you mimic or enhance the human’s knowledge or actions through technology? |
Test and evaluate the model | What new information do you know now? |
Deployment and integrate the model | What actions should you trigger with the new information? What needs human validation? |
Maintain the model | How has the data changed over time? Do the results reflect current reality? |