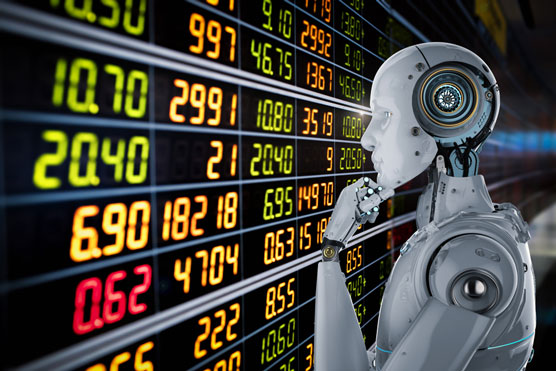
A KPMG report predicts that enterprises will increase their spending on intelligent automation from $12.4 billion in 2019 to $232 billion in 2025, almost 19 times as much in just seven years. A McKinsey report estimates that 20 percent of the cyclical tasks of a typical finance unit can be fully automated and almost 50 percent can be mostly automated.
Exploring the AI Pyramid
From all appearances, the industries typically served by professional services firms are in the early stages of a tectonic shift that will reverberate throughout the professional services industry. The initial shock will involve adopting new ways of organizing and delivering professional services, but the aftershocks could very well challenge the essence of what professional services firms deliver. The following figure shows the hierarchy of business complexity.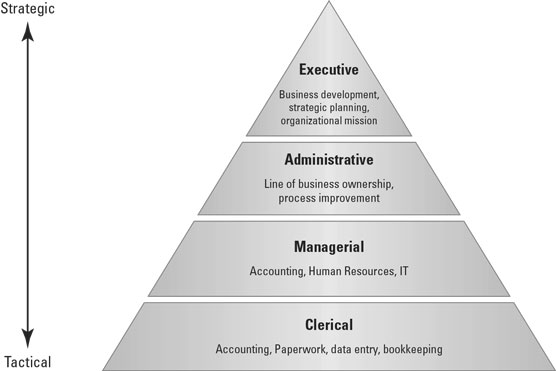
AI projects usually start at the base of the pyramid, where the goal is to save costs by optimizing manual process via human-machine collaboration. As the projects move up the pyramid, they move away from saving costs and focus on increasing revenue by making more informed decisions regarding existing lines of business or launching new lines of business.
In a real-life example, one cookware company uses home demonstrations to sell high-end pots and pans using internal financing. They brought in AI to replace a manual workflow based on rules and decision trees with a semi-automated process that streamlines the underwriting decision and reduces acquisition cost. This project was a tactical move to save money by making the process more efficient.
On the strength of that success, they moved up the pyramid. They used AI to analyze the historical behavior of accounts that underwriting declined and passed on to a third-tier lender. The model looked for common characteristics of borrowers that had been declined but got financing from the third-tier lender and didn’t default but paid it in full. They then applied the model to new loan applications to identify candidates who might not meet the traditional requirements for financing but were still a good risk. This project was a strategic move to expand their market to increase revenue.
To begin with, if AI doesn’t eventually replace the most fundamental tiers of service delivery, such as paper handling and data entry, it will at the very least optimize them to the point that they can be delivered by a significantly reduced staff through human-machine collaboration.
Or it could lead to an increase in staff by freeing up funds through increased efficiency, An Accenture report indicated that AI could boost employment levels by 10 percent if the rest of the world invested in AI and human-machine collaboration at the same level as the top performing 20 percent.
Professional services firms touch many industries, and just as technology matures and affects all industries, by necessity it affects how professional services firms engage their clients.
AI won’t replace core professional expertise, but it will make you more efficient and thus enable you to increase the value proposition for your clients. However, professionals who do embrace AI will replace those who don’t.
Climbing the AI Pyramid
The research tells us that enterprises across the board will increasingly turn to AI and big data to reduce costs and errors while improving efficiency and strategic planning. With a history of anticipating the needs of the market and then providing the services, you can use that knowledge to automate your own back-office processes and build on that experience to offer expanded services that relieve your clients of the heavy lifting of creating the architecture for an in-house or out-sourced AI initiative.Many firms focus on helping their clients automate routine tasks as a low-friction entry point with obvious time and cost savings. This simple application also serves as a platform for educating the client on the principles of AI and evaluating use cases for the best fit and results. With a proven win under their belt, clients are more receptive to expanding the role of AI and machine learning in their organizations, allowing them to introduce innovation and differentiation in their product and service offerings and to use the data to tackle tasks at higher levels of the pyramid.
By applying AI to your client’s environment, you can also increase your value to your clients by weaning them from reactively correcting when unexpected issues arise to forestalling common issues with preventative practices and ultimately to anticipating outcomes with predictive management.
As the application of AI to routine processes relieves employees from attending to mundane tasks, it also frees them to tackle more valuable and interesting tasks, thus enhancing their own career paths and adding more value for the clients.Another byproduct of the cycle of expanding automation upward through the tiers of the complexity pyramid is that, as the capabilities of artificial intelligence grow, the practices of your firm become more specialized until they are distilled to services that are beyond the touch of AI.
Or the singularity happens, whichever comes first.
But until that time, those who lean into innovation will gain the competitive advantage, but only if they incorporate continuous learning for their employees as a part of the business model.
Unearthing the Algorithmic Treasures
The uses for AI are as varied as the industries served by professional services firms.Healthcare
AI can quickly and economically acquire, classify, process, and route unstructured text to everyone in the information pipeline, increasing accessibility while lowering costs.Natural-language processing can extract targeted information from unstructured text such as faxes, clinical notes, intake forms, and medical histories, to improve end-to-end workflow. The process starts with data capture and classification, and then routes data and documents to the appropriate back-end systems, spotting exceptions, validating edge cases, and creating action items.
Content management
AI uses machine learning, text mining, and natural-language processing to process content, extracting concepts and entities, such as names, places, dates, and customized elements relevant to the business. AI then uses that information to create metadata and import it into a structured database, accelerating searches and data analysis. At the same time, the system automatically classifies the document based on its type and content and either assigns it to the next step in an automated workflow or flags it for review.Compliance
AI uses unstructured data mining, robotic process automation, statistical data aggregation, and natural-language processing to read and interpret compliance documents, interpret metadata, and identify roles and relationships, and then uses cognitive-process automation to deliver concise, actionable insights.AI uses supervised and unsupervised learning, natural-language processing, and intelligent segmentation to capture, analyze, and filter possible compliance violations to discard false positives that waste the time of compliance officers.
AI uses structured and unstructured data mining and natural-language processing to monitor internal and external records, documents, and social media to detect errors, violations, and trends, allowing the compliance department to be proactive and avoid costly penalties.
AI uses robotics-process automation, natural-language processing, and machine learning to identify potential violations of Know Your Customer (KYC) and Anti-Money Laundering (AML) regulations.
Law
AI uses text mining to process large pools of unstructured data, such as legal documents, emails, texts, and social media to identify key concepts, categorize content, detect subjectivity, isolate behavior patterns, discern the sentiment expressed in the content, and extract phrases and entities such as people, places, and things.AI uses supervised and unsupervised learning based on native or custom taxonomies to classify or characterize large volumes of documents and cull irrelevant documents as required in support of pre- and post-production activities, such as early case assessment and privilege detection.
AI uses machine learning and natural-language processing to analyze large amounts of textual content and distill it into short summaries and chronologies, which can display entity and concept trends over time, as well as behavioral patterns of persons of interest. The results can be integrated with data visualization to display the outcome in a consumable and intuitively understandable structure using interactive reports and dashboards.
Manufacturing
AI uses decision trees and neural networks to establish baseline requirements and then uses real-time data to reveal patterns and relationships to determine demand behavior, which drives optimized inventory levels and replenishment plans.AI uses text mining, data mining, and optimization-planning techniques to integrate suppliers and automate transactions to help clients understand their current business, address issues, and formulate strategies for improved performance. Clients can use supply-chain analytics to compare the performance of trading partners to operational and business metrics to make better decisions about their partnerships.
AI uses reinforcement learning to automate repetitive human processes. Robotic-process automation (RPA) combines analytics, machine learning and rules-based software to capture and interpret existing data-input streams to process a transaction, manipulate data, trigger responses, and communicate with other enterprise applications.
Oil and gas
AI uses predictive maintenance algorithms to achieve optimum uptime.AI uses IoT sensors and machine-learning algorithms to support data-driven decision-making and enable operational excellence for midstream processes, such as storing and transporting oil and gas.
AI uses text mining, natural-language processing, and machine learning to read legacy exploration and production data to optimize new construction and development projects.
AI uses text mining and machine learning to collect, combine, and assess data to improve operational performance, reduce cost, minimize risk, and accelerate time-to-production in well-site development. It also uses those techniques to boost health and safety and to improve environmental performance.