Data monetization
One trend in data that has taken hold is monetization. Monetizing data refers to how companies can utilize their domain expertise to turn the data they own or have access to into real, tangible business value or new business opportunities. Data monetization can refer to the act of generating measurable economic benefits from available data sources by way of analytics, or, less commonly, it may refer to the act of monetizing data services. In the case of data analytics, typically these benefits appear as revenue or cost savings, but they may also include market share or corporate market value gains.One could argue that data monetization for increased company revenue or cost savings is simply the result of being a data-driven organization. Though that argument isn’t totally wrong, company leaders are taking an increasing interest in the market to explore how data monetization can drive the innovation of entirely new business models in various different business segments.
One good example of how this process can work is when telecom operators sell data on the positions of rapidly forming clusters of users (picture the conclusion of a sporting event or a concert by the latest YouTube sensation) to taxi companies. This allows taxi cars to be available proactively in the right area when a taxi will most likely be needed. This is a completely new type of business model and customer base for a traditional telecom operator, opening up new types of business and revenues based on available data.Responsible AI
AI (artificial intelligence) has become a leader in data trends in recent years. Responsible AI systems are characterized by transparency, accountability, and fairness, where users have full visibility into which data is being used and how. It also assumes that companies are communicating the possible consequences of using the data. That includes both potential positive and negative impact.Responsible AI is also about generating customer and stakeholder trust based on following communicated policies and principles over time, including the ability to maintain control over the AI system environment itself.
Strategically designing your company´s data science infrastructure and solutions with responsible AI in mind is not only wise, but could also turn out to be a real business differentiator going forward. Just look at how the opposite approach, taken by Facebook and Cambridge Analytica, turned into a scandal which ended by putting Cambridge Analytica out of business. You might remember that Cambridge Analytica gained access to the private and personal information of more than 50 million Facebook users in the US and then offered tools that could then use that data to identify the personalities of American voters and influence their behavior. Facebook, rather than being hacked, was a willing participant in allowing their users' data to be used for other purposes without explicit user consent.
The data included details on users’ identities, friend networks, and “likes.” The idea was to map personality traits based on what people had liked on Facebook, and then use that information to target audiences with digital ads. Facebook has also been accused of spreading Russian propaganda and fake news which, together with the Cambridge Analytica incident, has severely impacted the Facebook brand the last couple of years. This type of severe privacy invasion has not only opened many people's eyes in terms of the usage of their data but also impacted the company brands.
Cloud-based data architectures
Cloud-based computing is a data trend that is sweeping the business world. More and more companies are moving away from on-premise-based data infrastructure investments toward virtualized and cloud-based data architectures. The driving force behind this move is that traditional data environments are feeling the pressure of increasing data volumes and are unable to scale up and down to meet constantly changing demands. On-premise infrastructure simply lacks the flexibility to dynamically optimize and address the challenges of new digital business requirements.Re-architecting these traditional, on-premise data environments for greater access and scalability provides data platform architectures that seamlessly integrate data and applications from various sources. Using cloud-based compute and storage capacity enables a flexible layer of artificial intelligence and machine learning tools to be added as a top layer in the architecture so that you can accelerate the value that can be obtained from large amounts of data.
Computation and intelligence in the edge
Let’s take a look at a truly edgy data trend. Edge computing describes a computing architecture in which data processing is done closer to where the data is created— Internet of Things (IoT) devices like connected luggage, drones, and connected vehicles like cars and bicycles, for example. There is a difference between pushing computation to the edge (edge compute) and pushing analytics or machine learning to the edge (edge analytics or machine learning edge).Edge compute can be executed as a separate task in the edge, allowing data to be preprocessed in a distributed manner before it’s collected and transferred to a central or semi-centralized environment where analytics methods or machine learning/artificial intelligence technologies are applied to achieve insights. Just remember that running analytics and machine learning on the edge requires some form of edge compute to also be in place to allow the insight and action to happen directly at the edge.
The reason behind the trend to execute more in the edge mainly depends on factors such as connectivity limitations, low-latency use cases where millisecond response times are needed to perform an immediate analysis and make a decision (in the case of self-driving cars, for example). A final reason for executing more in the edge is bandwidth constraints on transferring data to a central point for analysis. Strategically, computing in the edge is an important aspect to consider from an infrastructure-design perspective, particularly for companies with significant IoT elements.
When it comes to infrastructure design, it’s also worth considering how the edge compute and intelligence solutions will work with the centralized (usually cloud-based) architecture. Many view cloud and edge as competing approaches, but cloud is a style of computing where elastically scalable technology capabilities are delivered as a service, offering a supporting environment for the edge part of the infrastructure. Not everything, however, can be solved in the edge; many use cases and needs are system- or network-wide and therefore need a higher-level aggregation in order to perform the analysis. Just performing the analysis in the edge might not give enough context to make the right decision. Those types of computational challenges and insights are best solved in a cloud-based, centralized model.
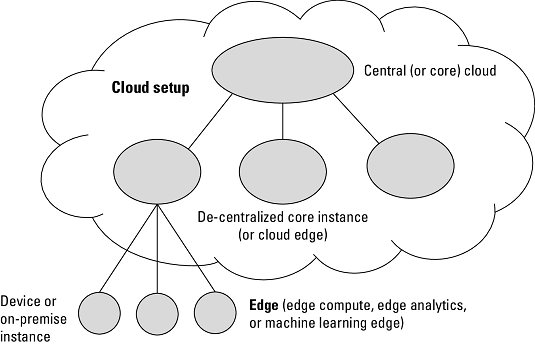
As you can see, the cloud setup can be done in a decentralized manner as well, and these decentralized instances are referred to as cloud-edge. For a larger setup on a regional or global scale, the decentralized model can be used to support edge implementations at the IoT device level in a certain country or to support a telecom operator in its efforts to include all connected devices in the network. This is useful for keeping the response time low and not moving raw data over country borders.
Digital twins
This particular trend in data will have you seeing double. A digital twin refers to a digital representation of a real-world entity or system — a digital view of a city's telecommunications network built up from real data, for example. Digital twins in the context of IoT projects is a promising area that is now leading the interest in digital twins. It’s most likely an area that will grow significantly over the next three to five years. Well-designed digital twins are assets that have the potential to significantly improve enterprise control and decision-making going forward.Digital twins integrate artificial intelligence, machine learning, and analytics with data to create living digital simulation models that update and change as their physical counterparts change. A digital twin continuously learns and updates itself from multiple sources to represent its near real-time status, working condition, or position.
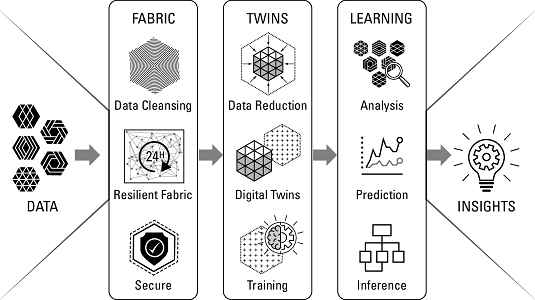
Digital twins are linked to their real-world counterparts and are used to understand the state of the system, respond to changes, improve operations, and add value. Digital twins start out as simple digital views of the real system and then evolve over time, improving their ability to collect and visualize the right data, apply the right analytics and rules, and respond in ways that further your organization's business objectives. But you can also use a digital twin to run predictive models or simulations which can be used to find certain patterns in the data building up the digital twin that might lead to problems. Those insights can then be used to prevent a problem proactively.
Adding automated abilities to make decisions based on the digital-twin concept of predefined and preapproved policies would be a great capability to add to any operational perspective — managing an IoT system such as a smart city, for example.
Blockchain
Blockchain is a trend in data that holds promise for future innovations. The blockchain concept has evolved from a digital currency infrastructure into a platform for digital transactions. A blockchain is a growing list of records (blocks) that are linked using cryptography. Each block contains a cryptographic hash of the previous block, a timestamp, and transaction data. By design, a blockchain is resistant to modification of the data. It’s an open and public ledger that can record transactions between two parties efficiently and in a verifiable and permanent way. A blockchain is also a decentralized and distributed digital ledger that is used to record transactions across many computers so that any involved record cannot be altered retroactively without the alteration of all subsequent blocks. The blockchain technologies offer a significant step away from the current centralized, transaction-based mechanisms and can work as a foundation for new digital business models for both established enterprises and start-ups. The image below shows how to use blockchain to carry out a blockchain transaction.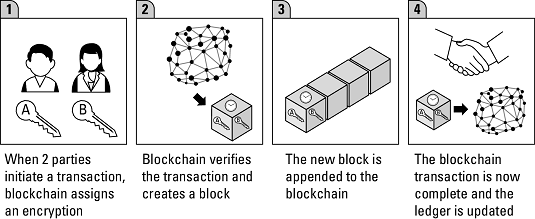
Although the hype surrounding blockchains was originally focused on the financial services industry, blockchains have many potential areas of usage, including government, healthcare, manufacturing, identity verification, and supply chain. Although blockchain holds long-term promise and will undoubtedly create disruption, its promise has yet to be proven in reality: Many of the associated technologies are too immature to use in a production environment and will remain so for the next two to three years.
Conversational platforms
Conversational AI is a form of artificial intelligence that allows people to communicate with applications, websites, and devices in everyday, humanlike natural language via voice, text, touch, or gesture input. For users, it allows fast interaction using their own words and terminology. For enterprises, it offers a way to build a closer connection with customers via personalized interaction and to receive a huge amount of vital business information in return. This image shows the interaction between a human and a bot.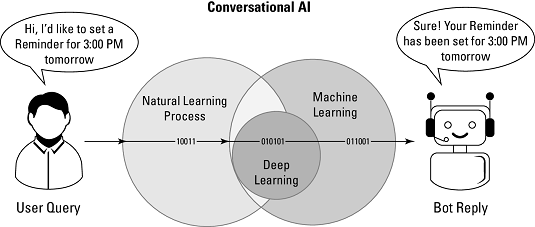
This trend in data will most likely drive the next paradigm shift in how humans interact with the digital world. The responsibility for translating intent shifts from humans to machines. The platform takes a question or command from the user and then responds by executing some function, presenting some content, or asking for additional input. Over the next few years, conversational interfaces will become a primary design goal for user interaction and will be delivered in dedicated hardware, core OS features, platforms, and applications.
Check out the following list for some potential areas where one could benefit from applying conversational platforms by way of bots:
- Informational: Chatbots that aid in research, informational requests, and status requests of different types
- Productivity: Bots that can connect customers to commerce, support, advisory, or consultative services
- B2E (business-to-employee): Bots that enable employees to access data, applications, resources, and activities
- Internet of Things (IoT): Bots that enable conversational interfaces for various device interactions, like drones, appliances, vehicles, and displays
Conversational platforms have now reached a tipping point in terms of understanding language and basic user intent, but they still aren’t good enough to fully take off. The challenge that conversational platforms face is that users must communicate in a structured way, and this is often a frustrating experience in real life. A primary differentiator among conversational platforms is the robustness of their models and the application programming interfaces (APIs) and event models used to access, attract, and orchestrate third-party services to deliver complex outcomes.